Big Data For Data Science
Module 6: Data Analysis for Big Data
Xingang (Ian) Fang
Outline
Products of data analysis
Data Analysis in Big Data Context
Machine learning
Deep learning
Products of data analysis
Reports and visualizations
Models
Statistical
Machine learning (including deep learning)
Optimized process/algorithm
New datasets
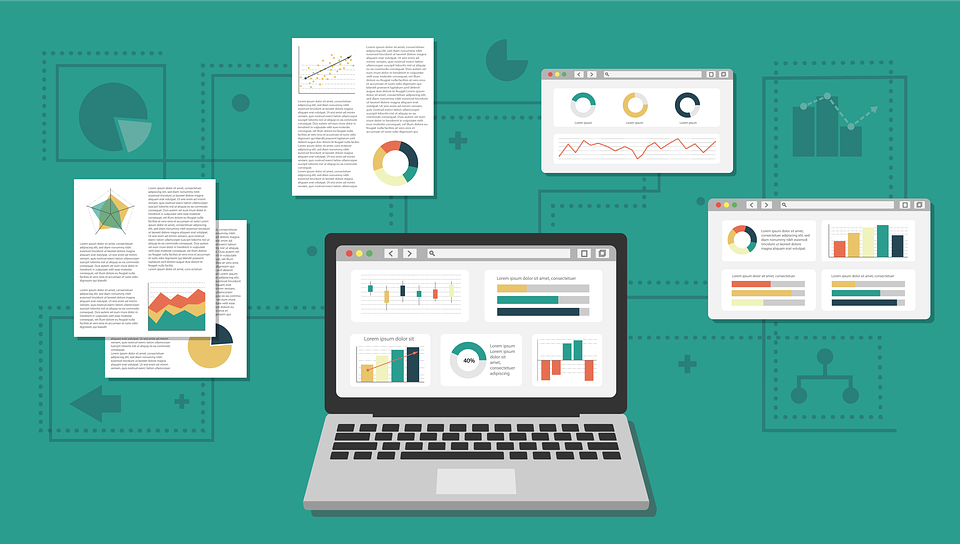
Data Analysis in Big Data Context
Two scenarios
Start big, finish small
Start big, finish big
Most data analysis methods only needs small data except for deep learning models
Statistical models
find trends and metrics
cannot model complex patterns
Machine learning: a subset of artificial intelligence that allows computers to learn from data and make predictions or decisions without being explicitly programmed.
Deep learning models (the only group of large models)
Most models are not “big”
Only large deep learning models will benefit from big volume of data
Readings:
![]()
Credit: Andrew Ng, CC BY-SA 4.0 <https://creativecommons.org/licenses/by-sa/4.0>, via Wikimedia Commons
Machine Learning vs Deep Learning
Machine Learning
Evolution: from rule-based to data-driven
Type of learning tasks
Traditional ML models
Libraries and frameworks
Deep Learning
Characteristics of DL
Comparison with traditional ML
DL models
Trending DL models
Libraries and frameworks
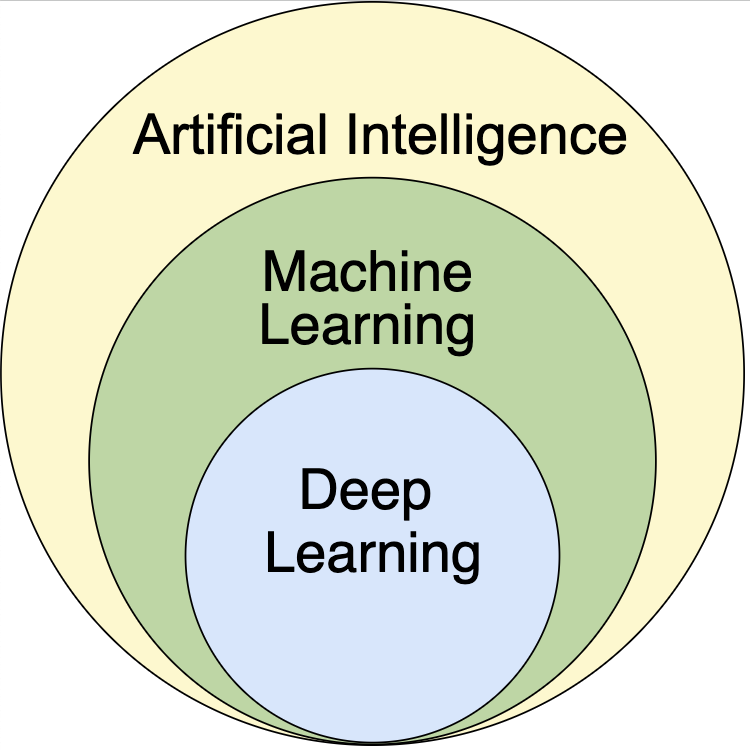
Credit: Lollixzc, CC BY-SA 4.0 <https://creativecommons.org/licenses/by-sa/4.0>, via Wikimedia Commons
Machine Learning
Evolution: from rule-based to data-driven
Rule-based: expert knowledge
Data-driven: data mining
Future: big data driven DL models
Type of learning tasks
Supervised learning: labeled data, learn to predict
Unsupervised learning: unlabeled data, learn to analyze and generate
Reinforcement learning: learn to make better decision with reward/punishment
Semi-supervised learning: a mix of labeled and unlabeled data
Self-supervised learning: unlabeled data, learn to predict itself
Ensemble learning: combine multiple models to improve performance
Transfer learning: learn from a related task and apply to a new task
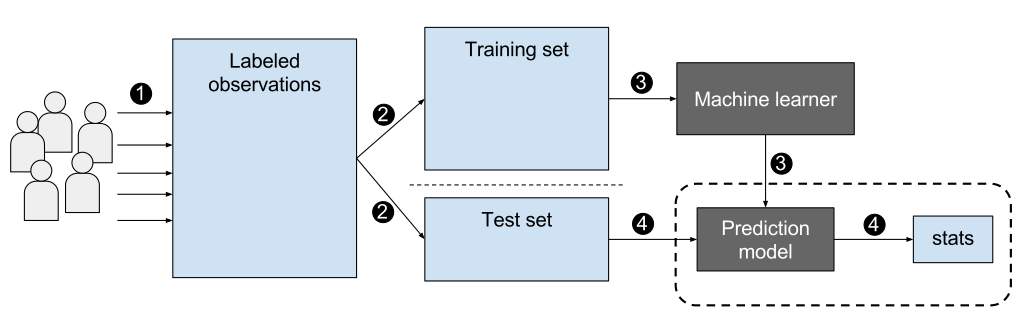
Credit: EpochFail, CC BY-SA 4.0 <https://creativecommons.org/licenses/by-sa/4.0>, via Wikimedia Commons
Traditional ML Models
Supervised learning
Linear Regression
Logistic Regression
Support Vector Machine
Unsupervised learning
K-means clustering
Principal Component Analysis (PCA)
Reinforcement learning
Q-learning
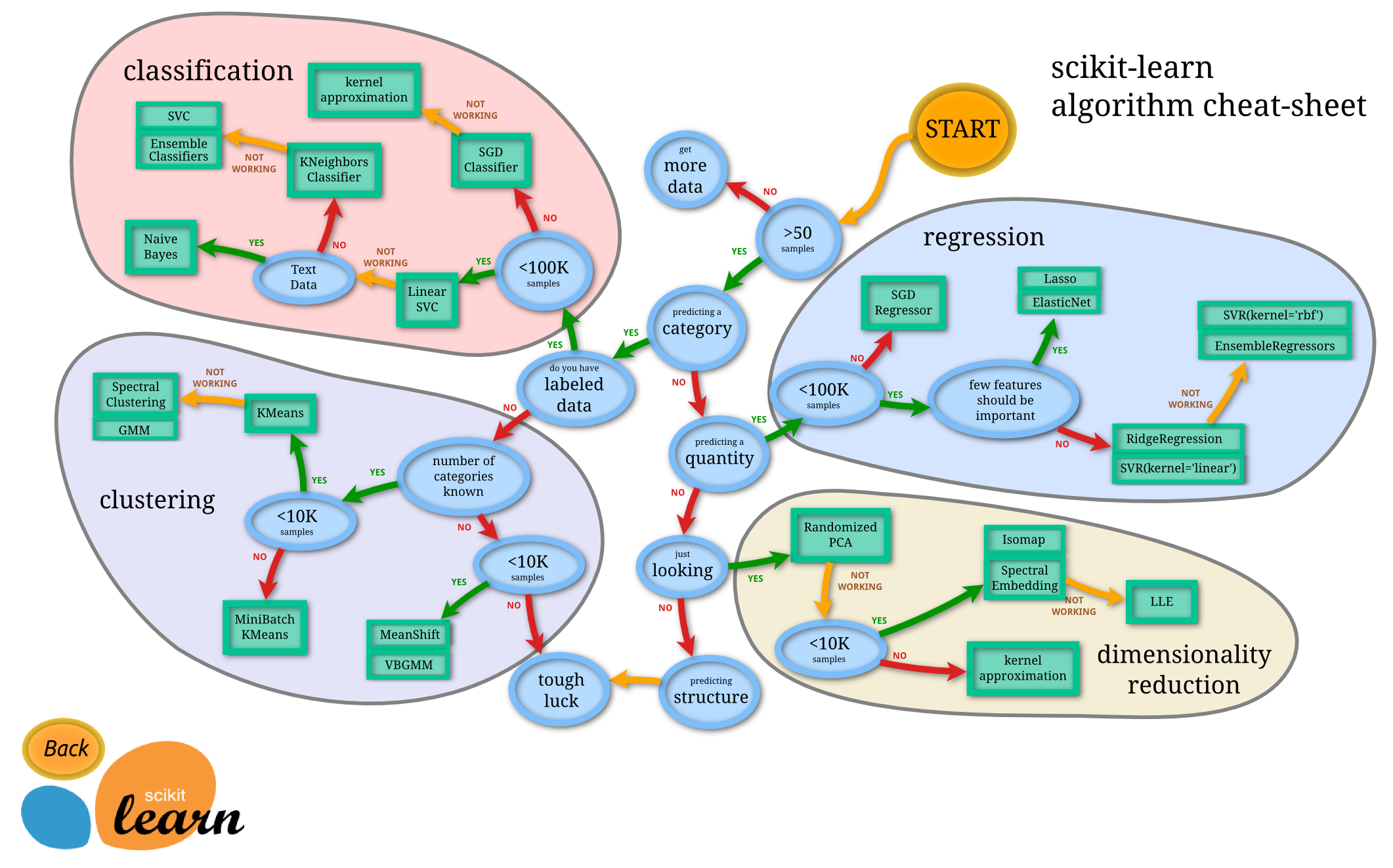
This informatics map only shows supervised and unsupervised models
Credit: https://scikit-learn.org/stable/tutorial/machine_learning_map/index.html
ML Libraries and Frameworks
Traditional ML
Scikit learning
R-studio
Spark MLLib
Visualization
Matplotlib
Seaborn
Plotly
Bokeh
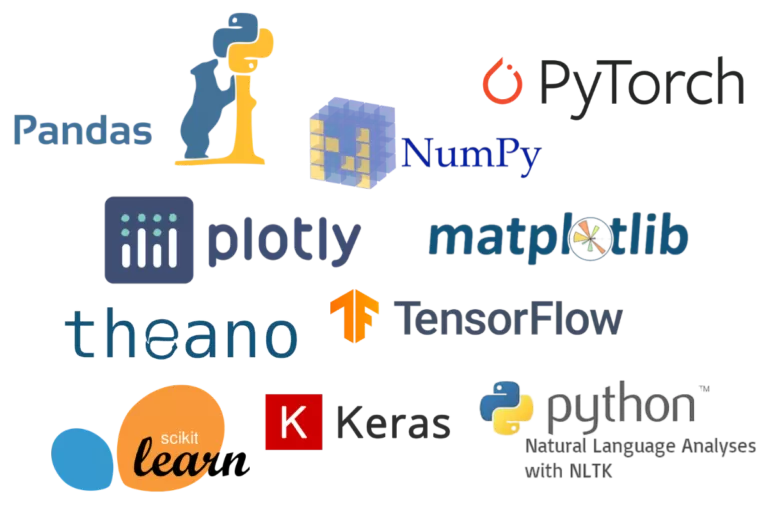
note: Image includes deep learning libraries
Credit: https://www.fireblazeaischool.in/blogs/python-libraries-for-machine-learning/
Deep Learning
Characteristics of DL
Ability to learn complex representations
Automatic feature extraction
Large amounts of data
Computationally intensive
Comparison with traditional ML
Data representation: learned feature vs hand-crafted feature
Complexity: huge number of parameters in DL models
Performance: Much better as data size increases
Data requirements: large amount of data
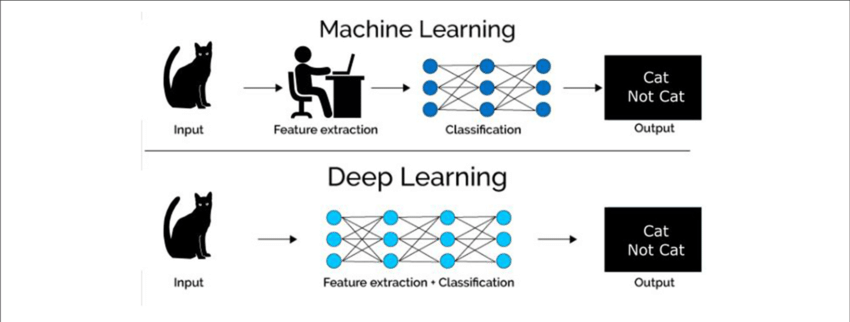
Credit: Asher, Clint, et al. “The Role of AI in Characterizing the DCM Phenotype.” Frontiers in Cardiovascular Medicine 8 (2021): 1986.
Deep Leaning Models
By learning task:
Supervised learning
Convolutional Neural Network (CNN)
Recurrent Neural Network (RNN)
Generative Adversarial Network (GAN)
Unsupervised learning
Autoencoder
Diffusion models
Generative Adversarial Network (GAN)
Reinforcement learning
Deep Q-Network (DQN)
By data type:
Computer vision
Convolutional Neural Network (CNN)
Recurrent Neural Network (RNN)
Generative Adversarial Network (GAN)
Diffusion models
Natural language processing
Transformer models
Matrix Factorization
Recurrent Neural Network (RNN)
Time-series data
Example: sound, speech, video, stock market data, etc.
Recurrent Neural Network (RNN)
Convolutional Neural Network (CNN)
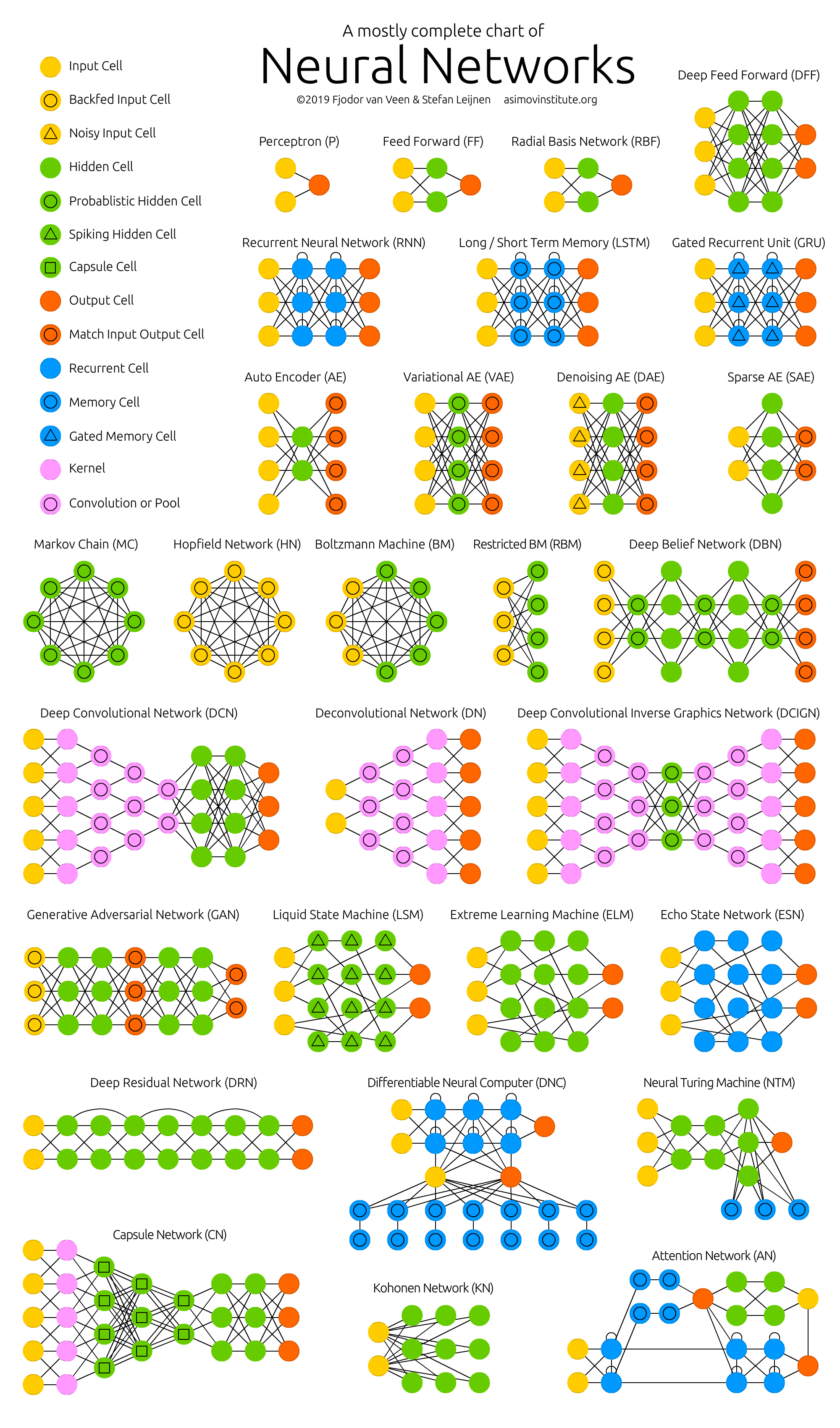
Trending DL Models
Large language models (LLM)
GPT family from OpenAI: GPT-3, GPT-4, ChatGPT
Google BERT, Palm, T5, Bard
Facebook RoBERTa, BigBird
BigScience BLOOM
Image generation models
OpenAI DALL-E
Stable Diffusion
MidJourney (possibly based-on stable diffusion)
Voice/Speech models
Google WaveNet, Universal Speech Model (USM)
OpenAI Whisper
DL Libraries and Frameworks
TensorFlow
PyTorch
Theano
Caffe 2
Chainer
Case Studies on Data Analysis for Big Data
Marr, Bernard; “Big Data Case Study Collection”, https://www.bernardmarr.com/img/bigdata-case-studybook_final.pdf
Oracle, “Top Big Data Analytics Use Cases”, https://www.oracle.com/a/ocom/docs/top-22-use-cases-for-big-data.pdf
Datamation.com, “How Big Data is Used by Netflix, AccuWeather, China Eastern Airlines, Etsy, and mLogica: Business Case Studies”, https://www.datamation.com/big-data/big-data-case-studies